Harnessing Natural Language Processing and Artificial Intelligence for Smarter Search
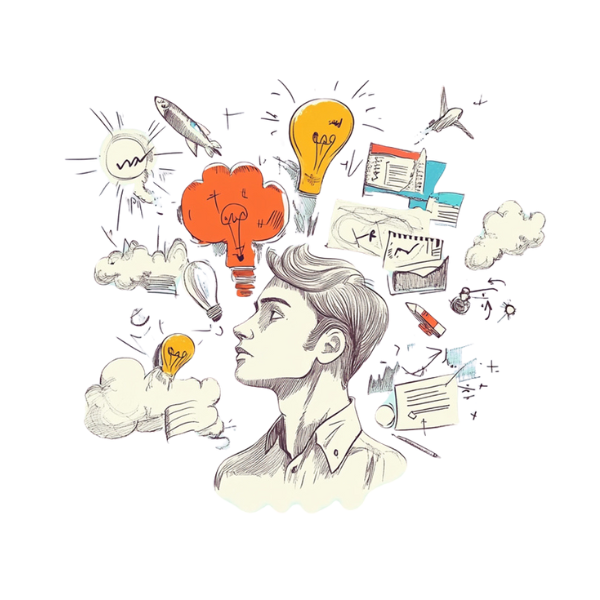
Over the years, search has become the primary way through which we interact with the internet, our computers, and other smart devices. The verb “to google” is now considered synonymous with looking up things online, and some university professors have even observed that their students increasingly fail to grasp the concept of organizing files into directories due to the ubiquity of search functionality. This evidence demonstrates the central role that search plays in our digital experience today.
Decades of research in information retrieval — the science of finding information — has fueled advancements in search that today form the backbone of a variety of diverse applications. Recently, however, new technologies have entered the scene. Advancements in Artificial Intelligence have taken the tech world by storm, as evidenced by the growing popularity of large language models (LLMs), such as OpenAI’s ChatGPT, Anthropic’s Claude, Meta’s Llama, and many more. These models, along with a series of significant breakthroughs in machine learning, have opened a whole new frontier in search, enabling ways to handle complex queries and questions in ways that were previously impossible.
In this article, we will survey some of the most important recent advances in search technology. After a brief introduction to traditional search methods, we will explore techniques that use advanced machine learning and natural language processing (NLP), focusing on vector and hybrid search specifically. Then, we will discuss how artificial intelligence can be integrated into search, and how emerging paradigms like retrieval augmented generation can contribute to its future.

Our focus throughout this article will be primarily on text search, that is, the task of finding relevant written documents given a user query. Search results can be considered relevant if they align well with the query and its intent. For example, assuming that the user is interested in visiting a cozy “Christmas Market”, relevant results for this term may include web pages with titles like "Top 10 Christmas Markets in Europe," "Local Christmas Market Events Near You," or "Guide to the Best Christmas Markets This Holiday Season". In contrast, pages titled “Stock Market Trends in December”, “Christmas Tree Farming Techniques”, or “The Best Supermarkets for Holiday Shopping”, would not be relevant.
Full-text Search
Many traditional search engines rely on full-text search, a family of algorithms that involve analyzing the input and candidate texts to provide related results. The exact details can vary from one algorithm to another, but full-text search solutions generally consist of two main steps:
- Indexing: analyzing documents to create a searchable index, often an inverted index. This results in a data structure that maps terms to documents containing them, which enables efficient retrieval.
- Retrieval: identifying the most relevant documents by applying a ranking function. The results are presented to the user ranked by their relevance to the input query.
Depending on the scale at which a search engine operates, the size of an index may be relatively limited (for example, the contents of all the pages from a small company’s website) or massive, up to hundreds of billions of webpages (e.g. Google’s search directory).
Text Processing for Full-text Search
Full-text search involves a number of text processing steps, which allow search engines to do more than simple keyword matching. For instance, when indexing data, modern search systems generally perform stemming. This is the process of breaking down words to their root form in order to diminish the influence of grammatical inflections on the results. The word “walking”, for example, can be reduced to the root “walk” and the suffix “-ing”. However, the word can take other forms as well, such as “walks”, “walked”, “walker”, etc. Stemming enables search engines to recognize that these are related and therefore treat them as such.
Another very common text processing step is stop word removal. This means that common but less meaningful words, such as articles, prepositions, and other grammatical particles are filtered out. Examples include "a", "an", "the", "is", "are", "was", "were", "in", "on", "at", and so on. Since these words occur very frequently across languages, their presence can decrease overall relevance, and are therefore usually not taken into account when searching.
Further text processing methods include tokenization (breaking down sentences into individual words), normalization (e.g. lowercasing everything), and lemmatization (similar to stemming, but reduces words to their dictionary forms, retaining more meaning). These methods contribute to the same goal: converting the contents of a document into a representation that is easier for search engines to work with.
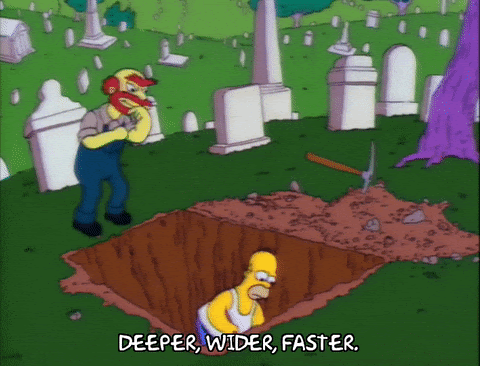
Retrieval
Once the documents have been processed and indexed, the engine is finally ready to perform retrieval. This is where search begins, making this the aspect of search systems that average users are most familiar with.
In order to retrieve relevant information, full-text search engines typically use a ranking function. These functions assign a score to each document in the index that represents how well it matches the input query. One of the most widely used functions of this kind is the BM25 algorithm (BM stands for “best matching”). BM25 builds on term-frequency methods that consider how frequently query terms appear both within each document and across the entire collection to determine how informative they are. In addition, the algorithm uses a set of tuneable parameters and weighting strategies to improve its ranking capabilities, making it one of the best-performing scoring mechanisms available today.
Thanks to the good balance it provides between performance and simplicity, BM25 remains one of the most popular ranking algorithms, despite having been an industry standard for decades. Moreover, it is used as the default scoring mechanism in many search applications, including popular open-source software, such as Apache Lucene and OpenSearch.

Full-text search may be a powerful tool in the search engineer’s toolbox, but it is most useful in cases where search results based on (near-)exact matches are sufficient. In other words, it might be perfect for search applications where users already have a more or less clear understanding of what they are looking for, and can therefore use keywords to retrieve the desired information. However, in applications that need to support a more open-ended kind of interaction with some content, traditional search engines often fail to meet expectations.
Challenges in Traditional Search
Human language is a complicated system, where words can be ambiguous and the same concept can be described using different expressions. People can phrase the same idea in various ways, and when searching, they often might not have a clear idea of exactly how to describe what they are looking for. What is more, the language we use to talk to each other doesn’t necessarily align well with how language is represented in computer systems.
In the context of search, the complexity of language presents a number of challenges. For example, traditional full-text search doesn’t handle synonyms or misspellings very well. Rather, these have to be explicitly coded to produce meaningful search results. If, for instance, a person is looking for information about obtaining a “construction permit” on a municipality’s website, but the latter only contains articles about getting a “building license”, they might not be able to find the answers they need. Furthermore, traditional search may not provide a broad enough context. The same person would probably also benefit from learning more about rules for construction in general, but may not be shown content relevant to this subject if the search results rely too heavily on the keywords “construction” and “permit” rather than providing a general overview as well.
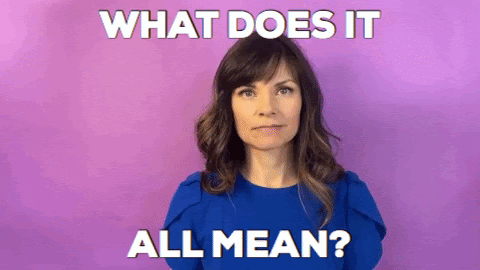
Semantic Search
To address these challenges, a new search paradigm — known as semantic search — has emerged. This family of retrieval algorithms provides an alternative to matching keywords. Semantic search aims to understand the intent and contextual meaning behind search queries, and can therefore deliver results that are more helpful and relevant.
In practice, semantic search is an umbrella term for a combination of various approaches that make traditional search more context-aware, and typically involves the following techniques:
- Query analysis: Using advanced NLP techniques, semantic search engines can get a better understanding of the input data. For example, Named Entity Recognition can be applied to identify proper nouns, such as names of places, people, and organizations.
- Query expansion: NLP can also be used to enhance queries by adding synonyms and related concepts to broaden the coverage of the search results. The query “driver’s license”, for instance, can be expanded to “driver’s license registration, application form, and renewal.”
- Knowledge graphs: These encode relationships between entities, which can improve results for queries that require factual knowledge. Relationships are often represented with triples, such as “:JamesCameron :directed :Titanic”.
- Personalization: In addition to understanding relationships between entities in text, semantic search systems can also leverage contextual information based on user behavior, such as history, location, or search preferences. Recommendations based on this kind of data contribute to the relevance of search results to individual users based on their individual needs.
Despite the considerable benefits semantic search brings to both search relevance and user experience, it often requires carefully engineered, domain-specific implementation. Semantic search developers must make sure, for example, that queries are analyzed and enhanced in ways that are relevant to the contents of the index against which queries are made. Dictionaries containing synonyms need to be constructed with this in mind - a word like “bank”, for instance, has a very different meaning in finance than in geography, where it may denote a riverbank rather than a financial institution. Similarly, knowledge graphs must be constructed with domain-specificity in mind to ensure accurate representation of entities and relationships.

Recognizing the limitations of technologies that require manually creating resources for representing linguistic and behavioral patterns, search engineers have turned to machine learning as a solution. This has led to a growing focus on algorithms that are capable of learning these representations themselves.
Machine learning (ML), a branch of artificial intelligence (AI), aims to enable computers to learn from vast amounts of data. Moreover, ML algorithms are built to make decisions and perform tasks autonomously, without human supervision. Advancements in machine learning have revolutionized various industries, from entertainment to medicine. For example, content recommendation systems on streaming platforms rely on ML models, in much the same way as disease diagnosis and drug discovery rely on algorithms trained on extensive datasets. And, of course, ML has been a key driver of the ongoing AI revolution.
Machine learning, of course, has also had a transformative impact in information retrieval. Search engines today increasingly leverage advanced ML techniques to provide more relevant and actionable results. Some of the ways in which ML has been influential in this field include techniques like text embeddings, vector and hybrid search, and retrieval augmented generation.
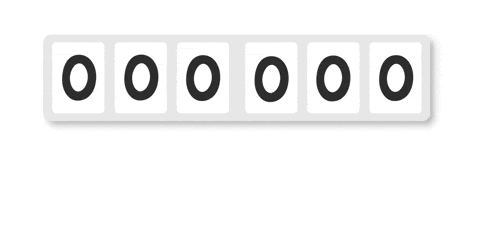
Vector Search
Representing natural language in a way that is easy for computers to understand has long been a challenge. However, a computational technique known as text embeddings — vector representations of words and sentences — has brought us one step closer to a possible solution. Grounded in distributional semantics, the idea that the building blocks of language, such as words and phrases, can be compared to each other based on the contexts in which they appear, these numerical representations make it possible to establish the semantic similarity between two pieces of text. Word vectors, for instance, can tell us that the words “France” and “Paris” relate to each other in the same way as “Germany” and “Berlin”. Vectors that encode longer chunks of text are also capable of showing such relationships. For example, they can determine that the following sentences are very similar in meaning:
- The capital of Denmark is a bike-friendly city blending history and modern design.
- Copenhagen boasts excellent cycling infrastructure and architecture that blends tradition with contemporary elements.
Vectors are learned using machine learning techniques from large amounts of data. The resulting model is essentially a computer program that converts text into a string of numbers that encodes information about each vector’s position and relationship to other vectors within a vector space. These vectors can then serve as a similarity metric across multiple dimensions of semantics, as embeddings of words and phrases that are close to each other in meaning are likely to be neighbors in the vector space. Thus, we can compare the vector of a search query to those of the documents in an index to find the ones that are most relevant semantically.
This process, known as nearest-neighbor search, brings various improvements over the strictly text-based methods we discussed earlier. For instance, vector search handles synonyms and variations in word choice much more efficiently. It is also resilient to typos and misspellings, which usually need to be corrected in full-text search systems. While these advantages are significant, the primary strength of vector search lies in how it provides a better overall semantic understanding of the search query and its intent, which works especially well for longer, natural-language searches.
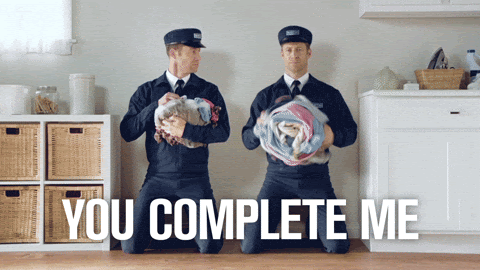
Hybrid Search
Despite the fundamental differences in how they work, full-text search and vector search should not be considered competing paradigms. In fact, combining them can pave the way toward a truly intelligent and robust search solution. On the one hand, keyword-based matching is lightweight, easy to implement, and works extremely well where exact matches and predictable outcomes are needed. On the other hand, vector search shines in applications that require a more complex semantic understanding of the input. Hybrid search, a combination of these two approaches, represents the best of both worlds.
Hybrid search can be implemented in different ways, but the most common approach is to run queries against both a full-text and a vector search engine and then combine the results using a fusion algorithm. Typically, the goal is to merge the results in such a way that both the keyword and the vector similarity scores contribute equally to the final ranking of documents, or at the very least, so that the strengths of both approaches are leveraged.
One of the most popular fusion algorithms, namely reciprocal rank fusion (RRF), was developed with exactly this purpose in mind. RRF merges documents from multiple different result sets by considering how highly they are ranked in each. The final ranking is obtained by summing up the reciprocal of the rank of each document in each result set, and then sorting accordingly. The algorithm does not require any manual tuning, and works even if the scores in the original rankings are on different scales. It is simple and computationally efficient, which explains its popularity in modern hybrid search implementations.Nowadays, there is a clear trend toward hybrid search becoming more and more of a standard within the search industry. Engineers and providers alike understand the importance of giving search semantic capabilities, while also retaining the strengths of traditional methods. Hybrid search functions as the most effective way to achieve this, which is why demand for this capability is growing rapidly.

Search may very well be the standard for looking for information online, but a list of blue links may not always be the best way to present it. In the era of chatbots and AI-assistants, integrating a large language model into a search system can enhance the overall user experience substantially.
LLMs are a powerful piece of technology, but unfortunately, they are often prone to generating incorrect or misleading information. Known as hallucinations, these issues can permanently undermine the public’s trust in systems that utilize such models. Solving hallucinations is an ongoing field of research, but for now, a technique called retrieval-augmented generation (RAG) can often mitigate the issue successfully.
In essence, RAG combines the accuracy and reliability of search with the conversational fluidity provided by generative AI models. At its core, it relies on a fairly simple idea. Given a search query and a set of relevant documents, the goal is for the language model to generate a helpful response. A family of applications where this paradigm can be useful is chatbots deployed on content-heavy websites. While in the past, chatbots would often rely on manually crafted rules and decision trees, RAG can simplify this process immensely. Given a robust semantic search engine integrated with an LLM, chatbots can answer all sorts of questions about a website’s content in a natural-sounding manner. Similarly, RAG is useful for generating summaries based on search results that are relevant and helpful given the input query.
Most importantly, perhaps, retrieval-augmented generation imposes certain limits on LLMs when it comes to what information they can include in their responses. This is why the approach is widely considered the most effective way to combat hallucinations today. Organizations need to make sure that if they use an LLM in their customer-facing applications, the responses the model gives are factually accurate and up-to-date. Thanks to the underlying search system, using RAG can make all the difference between potentially fabricated — and therefore inaccurate — information and reliable results coming from a verified source.

Throughout decades of research and development in the science of information retrieval, search has matured from simple keyword matching to a diverse variety of intelligent solutions. Technologies like hybrid search and retrieval augmented generation have shown not only that it is possible to combine natural language processing and the latest advancements in artificial intelligence to build smarter search, but also that this can be done without throwing away traditional and efficient methods. Today, AI is driving change in search at an unprecedented rate. Ultimately, the future is in our hands — and there is much to look forward to.
Article by András Aponyi